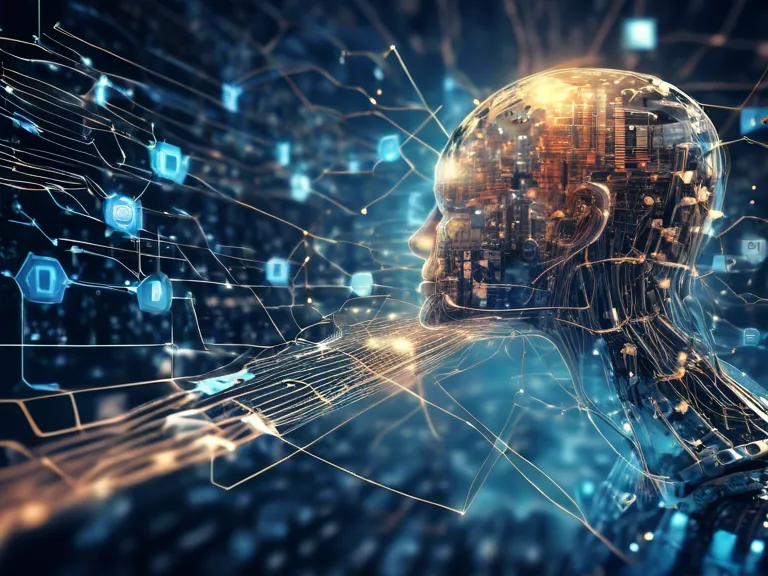
Introduction
Machine learning, a subset of artificial intelligence, is revolutionizing various industries by enabling computers to learn and improve from experience without explicit programming. As the field continues to evolve rapidly, it is crucial to stay updated on the latest trends and innovations shaping its future. In this article, we will delve into the emerging trends in machine learning that are poised to drive significant advancements in the coming years.
1. Explainable AI (XAI)
Explainable AI is gaining traction as organizations seek to understand and interpret the decisions made by machine learning models. This trend focuses on making AI algorithms more transparent and understandable to users. By providing insights into how algorithms arrive at a specific conclusion, XAI enhances trust, accountability, and regulatory compliance in machine learning applications.
2. Federated Learning
Federated learning addresses data privacy concerns by training machine learning models on decentralized data sources without the need to centralize the data. This approach allows multiple devices or servers to collaborate on model training while keeping data secure and private. Federated learning is particularly beneficial in scenarios where data cannot be easily shared due to privacy regulations or sensitive information.
3. AutoML and Automated Machine Learning
AutoML tools and platforms are simplifying the machine learning process by automating tasks such as data preprocessing, feature engineering, model selection, and hyperparameter tuning. These tools democratize machine learning by enabling users with limited expertise to build and deploy models efficiently. Automated machine learning accelerates the development cycle and empowers organizations to leverage AI capabilities without extensive manual intervention.
4. Edge Computing and Machine Learning at the Edge
Edge computing involves processing data closer to the source, reducing latency and enhancing real-time decision-making. Machine learning at the edge enables devices such as sensors, cameras, and IoT devices to perform AI tasks locally without relying on cloud infrastructure. This trend is crucial for applications requiring low latency, privacy, and bandwidth efficiency, such as autonomous vehicles, industrial IoT, and smart cities.
5. Continual Learning
Continual learning focuses on developing machine learning models that can adapt and learn incrementally from new data over time. Unlike traditional models that are trained on fixed datasets, continual learning algorithms evolve with changing environments and incoming data streams. This trend is essential for applications requiring continuous adaptation, such as personalized recommendations, anomaly detection, and adaptive control systems.
Conclusion
The future of machine learning is brimming with exciting possibilities, driven by these innovative trends and advancements. By staying abreast of these developments, organizations can harness the full potential of machine learning to drive innovation, improve decision-making, and unlock new opportunities across various domains. Embracing these trends will be instrumental in shaping a future where intelligent systems play a pivotal role in transforming industries and enhancing human experiences.
agoraguajara.com
amidstbooks.com
amoremagicallife.com
appspcdb.com
belatone.com
bistrotletranger.com
blackmountainfirearms.com
casollo.com
castlewoodlion.com
centromeninos.com
cubedentertainment.com
freestone-media.com
gargleblastrecords.com
geesent.com
glimmernews.com
iifingersbar.com
jean-samuel.com
kamakura-qs.com
liminal-order.com
mdgolden.com
monkeychop69.com
telegraphik.com
thmaniah8.com
ulf-mask.com
ulfbiotech.com
best-shoopping.com
blutreestudios.com
chateaumaplewood.com
dulichnguyenhung.com
fordlawfirmpllc.com
gandtblog.com
iwishihadapenguinfriend.com
kbresre.com
kimihime.com
manggih.com
marcelogoes.com
mustlovedanesrescue.com
nangluong-mattroi.com
nicolemidwest.com
paulinevknguyen.com
seleneboutiquesorrento.com
shanghai-culture.com
singturmg.com
thefantasyguide.com
theholygeek.com
thepressshopnyc.com
transportreloaded.com
whereeverigo.com
askchrisknight.com
askchristopherknight.com
berkshirefoodjournal.com
cchirosw.com
coastalcottagesnl.com
destinationsite.com
finulldraft.com
gardenstatepaintingnj.com
impacthound.com
inneddi.com
itazurakoneko.com
judyforaz.com
localcarsnow.com
lovedayscinema.com
matiqistiqomahsambas.com
meetborofive.com
morskicovik.com
naturesrewardphotography.com
regenerativeresorts.com
restaurant-nashvilletn.com
scoopsicecreamtruck.com
simmonsmotorworks.com
trinityeco-tours.com
uncoverstudios.com
wong-multimedia.com
123exp-government.com
abozymes.com
atsheatingandair.com
bemjavim.com
caseintrend.com
cdnxbvn.com
charangolibre.com
denis-latin.com
frugal-science.com
fukuoka-kouyaren.com
hanabisou.com
herculessportcenter.com
highvoltageloja.com
hlwstoryboard.com
juderabig.com
leauxandbehold.com
melurix-next.com
moggiechicken.com
mytrendysins.com
nativeskinonline.com
ninethreestudio.com
pcmwereld.com
pichinkufibers.com
primerautobody.com
salmanhatta.com
shalevbrosh.com
sosorryrecords.com
sportsone-egy.com
wofome.com
ycadeau.com
abalawpc.com
airportpawnmiami.com
alchemycollaborative.com
blackfigdesigns.com
cubemedica.com
deliciousyarns.com
denledamtrancaocap.com
denledpanelcaocap.com
futebolrico.com
honsyuji.com
jayasupranaschool.com
jstact.com
kikichaos.com
knuckleupmartialarts.com
lavi-furniture.com
maisonsecomatiq.com
neighbors-construction.com
nspointers.com
opticiwear.com
richouli.com
soddydaisyhealthcare.com
soultalkmagazine.com
studywithpmouy.com
swordfightsandstarrynights.com
tamatur-bali.com
thepainreliefsecret.com
townhouselinens.com
vchainstore.com
vormaro.com
yukonlodgeforsale.com
airkoolny.com
an-geeke.com
bajka3.com
bertinoargenti.com
birolkitabevi.com
burikoland.com
emeraldseamless.com
floridanativecharters.com
iptvsportt.com
jupiterboutique.com
kateronline.com
kustomkoachrv.com
lademeuredufondateur.com
lucianamurta.com
luckydelaware.com
luckyindiana.com
lujannavas.com
mike4rep.com
milioshairsalon.com
movingrestaurantequipment.com
openseedvault.com
retrogradetours.com
revistaresiduos.com
roughandreadygrange.com
therusalka.com
toxnfill3.com
valleymaintenancelandscape.com
violetorlandi.com
wanpaku-golf.com
zinkdental.com
suryacctv.com
tuvannugioi.com
mandalynmusic.com
wireless-reviews.com
tussingblockwatch.com
greenteaphotography.com
jennierooney.com
vietamreview.net
kyavar.com
market-truth.com
alexandregaurier.com
omegagadget.com
banditosla.com
nursememama.com
oyunbilim.com
acme-nuclear.com
agilityimap.com
akikcombong.com
anniesmysteries.com
bflofoodie.com
brandzonestudios.com
cacemar.com
daperezlaw.com
denvyautomation.com
eugeneband.com
factory-eshop.com
florentdumas.com
hishaywireless.com
in2-signs.com
kuwekeza-holdings.com
mitaniya-ltd.com
mixfoure.com
mobilitypluspro2.com
moipravila.com
montreal-business-kit.com
mortiseandmiter.com
nextdigitaldental.com
nurdalilahputri.com
oem-phoneaccessories.com
palmbeachestepona.com
precavida.com
roscoeandetta.com
scriptsnmacros.com
sringserver.com
thecustomfairy.com
withlovefromangela.com
applebyandwood.com
auzigog.com
eac-w.com
homesbyelevation.com
nihilismforoptimists.com
slavonkandhortus.com
thekoreanpolitics.com
turningpointpt.com
val-up.com
wakansen.com
3dideation.com
achilles-fire.com
banatelhalal.com
biyografirehberi.com
bohams.com
comisiondeestudios.com
cooride-net.com
danayumul.com
ecadecom.com
edwardscornerfarmersmarket.com
ekspresif.com
ellajmae.com
ginroecooks.com
gracefueled.com
hightidekitchen.com
jeroenswolfs.com
marthalott.com
mollybroekman.com
mpthoidai.com
plumfashionpr.com
racktents.com
solzapower.com
southcoastbehavioralhealth.com
the101bali.com
thearguide.com
theartistfia.com
thefitnesswire.com
thelivelihoodproject.com
thelynndentonagency.com
wilkespoolsnspas.com
wjwatson.com
drinkganbei.com
mendenhallnews.com
nathaliemoliavko-visotzky.com
nationalinfertilityday.com
wide-aware.com
ashleymodernfurniture.com
babylonbusinessfinance.com
charliedewhirst.com
christianandmilitaryhats.com
hypnosisoneonone.com
icelandcomedyfilmfestival.com
kayelam.com
mlroadhouse.com
mumpreneursonline.com
posciesa.com
pursweets-and.com
rgparchive.com
therenegadehealthshow.com
travelingbitz.com
yutakaokada.com
22fps.com
aarondgraham.com
essentialaustin.com
femdotdot.com
harborcheese.com
innovar-env.com
mercicongo.com
oabphoto.com
pmptestprep.com
rmreflectivevest-jp.com
tempistico.com
filmintelligence.org
artisticbrit.com
avataracademyagency.com
blackteaworld.com
healthprosinrecovery.com
iancswanson.com
multiversecorpscomics.com
warrenindiana.com
growthremote.com
horizonbarcelona.com
iosdevcampcolorado.com
knoticalpr.com
kotaden.com
la-scuderia.com
nidoderatones.com
noexcuses5k.com
nolongerhome.com
oxfordcounselingcenter.com
phytacol.com
pizzaropizza.com
spotlightbd.com
tenbags.com
thetravellingwilbennetts.com
archwayintl.com
jyorganictea.com
newdadsplaybook.com
noahlemas.com
qatohost.com
redredphoto.com
rooms4nhs.com
seadragonenergy.com
spagzblox.com
toboer.com
aumantvmuseum.com
beyondausten.com
citylabstudio.com
diskonio.com
drinkcf.com
eft-dongle.com
emilymeganphotography.com
evolveathleticclub.com
godleystationvet.com
hirochanweb.com
homeonefurniture.com
ifiwasastylist.com
lacantinepopup.com
liriklagubatak.com
lo-ko.com
mensagenseatividades.com
myway-zeus.com
nevadadec.com
nokarikhabar.com
nuuuki.com
quenchpad.com
sckyrock.com
tindunghanoi.com
tradeshows-biz.com
wikimuzik.com