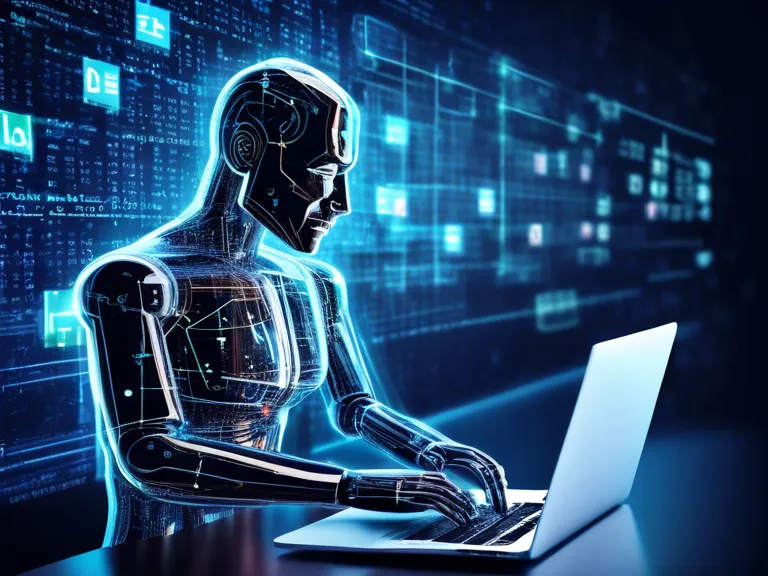
Machine learning applications in payment fraud detection have revolutionized the way financial institutions and businesses combat fraudulent activities. By leveraging advanced algorithms and data analysis techniques, machine learning models can identify patterns, anomalies, and trends that traditional rule-based systems may overlook. In this article, we will explore the key concepts, benefits, challenges, and real-world applications of machine learning in payment fraud detection.
Understanding Payment Fraud Detection
Payment fraud refers to any unauthorized or fraudulent activity that involves the use of payment methods to steal money or sensitive information. Common types of payment fraud include credit card fraud, identity theft, account takeover, and phishing scams. Detecting and preventing payment fraud is crucial for protecting consumers, businesses, and financial institutions from financial losses and reputational damage.
Benefits of Machine Learning in Payment Fraud Detection
Machine learning offers several advantages over traditional fraud detection methods, including:
Improved Accuracy: Machine learning algorithms can analyze large volumes of data in real-time to detect subtle patterns and anomalies that may indicate fraudulent behavior.
Adaptability: Machine learning models can adapt and evolve based on new data and emerging fraud trends, enhancing their ability to detect evolving fraud schemes.
Automation: Machine learning enables automated decision-making processes, reducing the need for manual intervention and speeding up the fraud detection process.
Scalability: Machine learning systems can scale to handle large volumes of transactions, making them suitable for businesses of all sizes.
Challenges in Implementing Machine Learning for Payment Fraud Detection
While machine learning offers significant benefits, there are also challenges associated with implementing these technologies for payment fraud detection:
Data Quality: Machine learning models require high-quality data to produce accurate results. Incomplete or inaccurate data can lead to false positives or false negatives.
Model Interpretability: Some machine learning algorithms are complex and difficult to interpret, making it challenging to understand how decisions are made.
Adversarial Attacks: Fraudsters may try to manipulate machine learning models by feeding them misleading data to evade detection.
Regulatory Compliance: Financial institutions must ensure that their machine learning models comply with data privacy and regulatory requirements, such as GDPR and PCI DSS.
Real-World Applications of Machine Learning in Payment Fraud Detection
Numerous financial institutions and payment processors are leveraging machine learning technologies to enhance their fraud detection capabilities. Some common applications include:
Anomaly Detection: Machine learning models can identify unusual patterns or behaviors in transaction data that may indicate fraudulent activity.
Behavioral Analysis: By analyzing customer behavior and transaction history, machine learning models can detect deviations from normal patterns and flag potentially fraudulent transactions.
Network Analysis: Machine learning algorithms can analyze complex networks of transactions to identify suspicious connections or relationships between accounts.
Predictive Analytics: Machine learning can be used to predict future fraud risks based on historical data and trends, enabling proactive fraud prevention measures.
In conclusion, machine learning plays a crucial role in enhancing payment fraud detection capabilities by enabling real-time analysis, automation, and adaptability. While there are challenges to overcome, the benefits of using machine learning in fraud detection far outweigh the drawbacks. As fraudsters become more sophisticated, financial institutions and businesses must continue to innovate and leverage advanced technologies to stay ahead of evolving fraud threats.
askchrisknight.com
askchristopherknight.com
berkshirefoodjournal.com
cchirosw.com
coastalcottagesnl.com
destinationsite.com
finulldraft.com
gardenstatepaintingnj.com
impacthound.com
inneddi.com
itazurakoneko.com
judyforaz.com
localcarsnow.com
lovedayscinema.com
matiqistiqomahsambas.com
meetborofive.com
morskicovik.com
naturesrewardphotography.com
regenerativeresorts.com
restaurant-nashvilletn.com
scoopsicecreamtruck.com
simmonsmotorworks.com
trinityeco-tours.com
uncoverstudios.com
wong-multimedia.com
123exp-government.com
abozymes.com
atsheatingandair.com
bemjavim.com
caseintrend.com
cdnxbvn.com
charangolibre.com
denis-latin.com
frugal-science.com
fukuoka-kouyaren.com
hanabisou.com
herculessportcenter.com
highvoltageloja.com
hlwstoryboard.com
juderabig.com
leauxandbehold.com
melurix-next.com
moggiechicken.com
mytrendysins.com
nativeskinonline.com
ninethreestudio.com
pcmwereld.com
pichinkufibers.com
primerautobody.com
salmanhatta.com
shalevbrosh.com
sosorryrecords.com
sportsone-egy.com
wofome.com
ycadeau.com
abalawpc.com
airportpawnmiami.com
alchemycollaborative.com
blackfigdesigns.com
cubemedica.com
deliciousyarns.com
denledamtrancaocap.com
denledpanelcaocap.com
futebolrico.com
honsyuji.com
jayasupranaschool.com
jstact.com
kikichaos.com
knuckleupmartialarts.com
lavi-furniture.com
maisonsecomatiq.com
neighbors-construction.com
nspointers.com
opticiwear.com
richouli.com
soddydaisyhealthcare.com
soultalkmagazine.com
studywithpmouy.com
swordfightsandstarrynights.com
tamatur-bali.com
thepainreliefsecret.com
townhouselinens.com
vchainstore.com
vormaro.com
yukonlodgeforsale.com
airkoolny.com
an-geeke.com
bajka3.com
bertinoargenti.com
birolkitabevi.com
burikoland.com
emeraldseamless.com
floridanativecharters.com
iptvsportt.com
jupiterboutique.com
kateronline.com
kustomkoachrv.com
lademeuredufondateur.com
lucianamurta.com
luckydelaware.com
luckyindiana.com
lujannavas.com
mike4rep.com
milioshairsalon.com
movingrestaurantequipment.com
openseedvault.com
retrogradetours.com
revistaresiduos.com
roughandreadygrange.com
therusalka.com
toxnfill3.com
valleymaintenancelandscape.com
violetorlandi.com
wanpaku-golf.com
zinkdental.com
suryacctv.com
tuvannugioi.com
mandalynmusic.com
wireless-reviews.com
tussingblockwatch.com
greenteaphotography.com
jennierooney.com
vietamreview.net
kyavar.com
market-truth.com
alexandregaurier.com
omegagadget.com
banditosla.com
nursememama.com
oyunbilim.com
acme-nuclear.com
agilityimap.com
akikcombong.com
anniesmysteries.com
bflofoodie.com
brandzonestudios.com
cacemar.com
daperezlaw.com
denvyautomation.com
eugeneband.com
factory-eshop.com
florentdumas.com
hishaywireless.com
in2-signs.com
kuwekeza-holdings.com
mitaniya-ltd.com
mixfoure.com
mobilitypluspro2.com
moipravila.com
montreal-business-kit.com
mortiseandmiter.com
nextdigitaldental.com
nurdalilahputri.com
oem-phoneaccessories.com
palmbeachestepona.com
precavida.com
roscoeandetta.com
scriptsnmacros.com
sringserver.com
thecustomfairy.com
withlovefromangela.com
applebyandwood.com
auzigog.com
eac-w.com
homesbyelevation.com
nihilismforoptimists.com
slavonkandhortus.com
thekoreanpolitics.com
turningpointpt.com
val-up.com
wakansen.com
3dideation.com
achilles-fire.com
banatelhalal.com
biyografirehberi.com
bohams.com
comisiondeestudios.com
cooride-net.com
danayumul.com
ecadecom.com
edwardscornerfarmersmarket.com
ekspresif.com
ellajmae.com
ginroecooks.com
gracefueled.com
hightidekitchen.com
jeroenswolfs.com
marthalott.com
mollybroekman.com
mpthoidai.com
plumfashionpr.com
racktents.com
solzapower.com
southcoastbehavioralhealth.com
the101bali.com
thearguide.com
theartistfia.com
thefitnesswire.com
thelivelihoodproject.com
thelynndentonagency.com
wilkespoolsnspas.com
wjwatson.com
drinkganbei.com
mendenhallnews.com
nathaliemoliavko-visotzky.com
nationalinfertilityday.com
wide-aware.com
ashleymodernfurniture.com
babylonbusinessfinance.com
charliedewhirst.com
christianandmilitaryhats.com
hypnosisoneonone.com
icelandcomedyfilmfestival.com
kayelam.com
mlroadhouse.com
mumpreneursonline.com
posciesa.com
pursweets-and.com
rgparchive.com
therenegadehealthshow.com
travelingbitz.com
yutakaokada.com
22fps.com
aarondgraham.com
essentialaustin.com
femdotdot.com
harborcheese.com
innovar-env.com
mercicongo.com
oabphoto.com
pmptestprep.com
rmreflectivevest-jp.com
tempistico.com
filmintelligence.org
artisticbrit.com
avataracademyagency.com
blackteaworld.com
healthprosinrecovery.com
iancswanson.com
multiversecorpscomics.com
warrenindiana.com
growthremote.com
horizonbarcelona.com
iosdevcampcolorado.com
knoticalpr.com
kotaden.com
la-scuderia.com
nidoderatones.com
noexcuses5k.com
nolongerhome.com
oxfordcounselingcenter.com
phytacol.com
pizzaropizza.com
spotlightbd.com
tenbags.com
thetravellingwilbennetts.com
archwayintl.com
jyorganictea.com
newdadsplaybook.com
noahlemas.com
qatohost.com
redredphoto.com
rooms4nhs.com
seadragonenergy.com
spagzblox.com
toboer.com
aumantvmuseum.com
beyondausten.com
citylabstudio.com
diskonio.com
drinkcf.com
eft-dongle.com
emilymeganphotography.com
evolveathleticclub.com
godleystationvet.com
hirochanweb.com
homeonefurniture.com
ifiwasastylist.com
lacantinepopup.com
liriklagubatak.com
lo-ko.com
mensagenseatividades.com
myway-zeus.com
nevadadec.com
nokarikhabar.com
nuuuki.com
quenchpad.com
sckyrock.com
tindunghanoi.com
tradeshows-biz.com
wikimuzik.com